Choose to both consider data and challenge bias
↓ NextWhen it comes to taking on the complex issues affecting marginalized people, understanding historical facts and nuanced insights is key.
↓ NextBut taking data points as the final word on an issue, without examining their context or origin, can do almost as much harm as the problems themselves.
↓ NextEven the most rigorous data sets can have bias baked into them. Some biases are contained within historic approaches that were intentionally harmful to marginalized communities, and can still be today. Sometimes, we overlook the systems that impact the data. Still other times, we read or compile data with the human biases we all possess.
↓ NextBiased data can create dangerous, reinforcing assumptions about issues and communities. Whether it’s a credit rating that doesn’t account for systemic wealth gaps, or an algorithm using biased insights to predict criminal activity, data is not objective. Strategies that leverage data points without critique are off to an over-simplified start.
↓ Next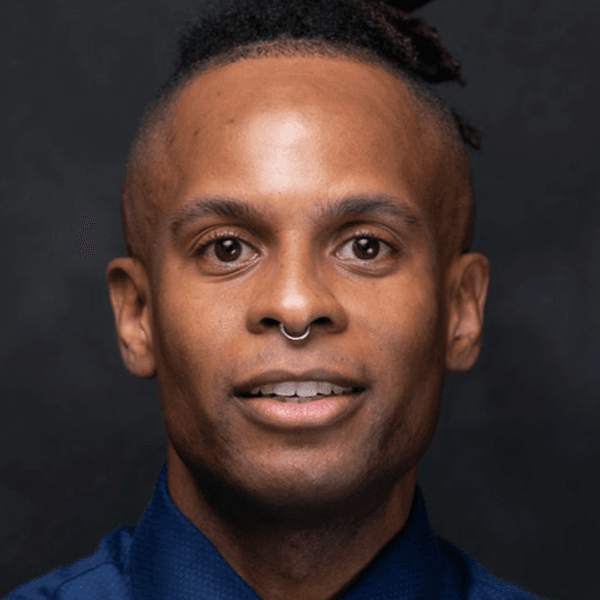
Data can be powerful, and even more so when surrounded by the right perspectives.
In our field, no one would dare argue against the necessity of grounding everything that we do in data. “follow the data” is the rallying cry of those who want to be perceived as logical, clear-eyed, and objective. Anyone who raises a precautionary concern about how data is collected, analyzed, or evaluated runs the risk of being branded as too emotional and irrational to achieve real, tangible change.
However, appeals to “follow the data” do not always reflect scientific fact or the laws of nature that govern the world we all share. Often “the data” is a rhetorical device used to grant narrative authority to certain perspectives—those that confirm preconceived ideas despite being underpinned by weak study designs, arbitrary assumptions, or erroneous math that should alert audiences to interpret the findings cautiously. The intent is to shame people for straying from the supposed experts’ superior rationality, and ultimately to portray any demand for change to the status quo as fringe, conspiratorial, and anti-scientific.
Data only tells us what happened—not why, how, or what the significance is. For data to tell a story or convey key insights about social impact, we must add context and structure—and be transparent about the choices that are made in how those additions are shaped or integrated.
Data can tell us many things about the realities of issues like the homeownership gap between Black and White communities in the U.S. It can’t explain to us the motivations and rationales of minority communities who must navigate racial bias in their interactions with the broad range of actors within the housing sector, or recognize the validity of the knowledge held by those who are most affected by the issue. The way forward requires a commitment to data equity and transformative accountability as well as data quality and rigorous methodology.
For those in the social impact field, especially evaluators, data scientists, and researchers, it is imperative that we go into our work with an awareness of the biases that shape how we model, sample, analyse, interpret, select methodologies and even perform basic mathematical operations like calculating an average. Start by identifying and understanding each place in your work where a biased, hierarchical, or oppressive worldview could skew our ideas of what data is most necessary or valuable to prove a point, drive a conclusion, or make a decision.
Demonstrate that the data you are producing is reliable and most importantly created in a process that brings awareness, intentionality, and meaningfully embeds a strong commitment to equity at each step. Level the playing field between technical experts, stakeholders, and impacted communities in deciding what data or information is most integral to your work, and how you plan to collect or analyze it.
Don’t prioritize the most privileged people in the way that your findings are communicated—match your communication content, design, medium, and accessibility to reflect the equity you want to see in your work and in the world, as well as uplifting the expertise of the most marginalized. We should be engaging with data in a way that serves impacted communities, instead of extracting or using it in ways that undermine their needs.
When it comes to taking on the complex issues affecting marginalized people, understanding historical facts and nuanced insights is key.